The Artificial System of Classification: Foundations of Early Taxonomy
- I. Introduction
- II. Historical Context of Early Taxonomy
- III. The Principles of Artificial Classification
- IV. Notable Artificial Classification Systems
- V. Comparison with Other Classification Systems
- VI. Advantages of Artificial Classification
- VII. Case Studies
- VIII. Modern Relevance of Artificial Classification
- References:
I. Introduction
The development of early taxonomy was profoundly influenced by the establishment of artificial systems of classification, which structured the complex diversity of the natural world into manageable categories. This organizing principle was driven by a necessity to communicate and comprehend the myriad forms of life that inhabited the planet. Such classifications were not arbitrary; they reflected a systematic approach, articulating relationships among organisms that facilitated scientific inquiry and discovery. The advent of seminal thinkers, like Carl Linnaeus, marked a pivotal moment in this evolution as they pioneered methods that combined observational rigor with hierarchical organization. Moreover, these artificial systems exemplified an intersection of philosophy and empiricism, urging scientists to categorize life based on shared characteristics rather than solely on superficial traits. As these systems solidified, they laid the groundwork for modern biological classification, evolving from simple categorizations to more intricate frameworks that continue to shape the study of biodiversity today.
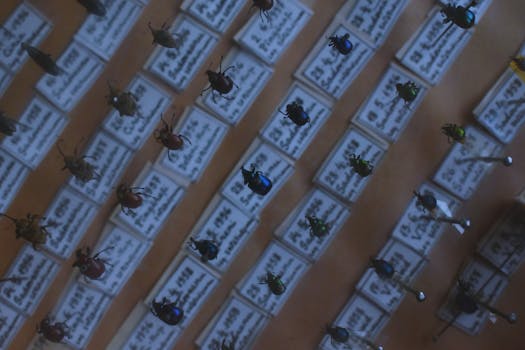
A. Definition and Overview
The exploration of artificial systems of classification has roots in the early endeavors of taxonomy, where the need for systematic organization became apparent. Fundamental to understanding early taxonomy is the recognition that initial attempts to categorize organisms were often based on observable attributes, leading to artificial systems—classification approaches that prioritize convenience over natural relationships. As noted in the literature, these systems laid the groundwork for contemporary applications, where advances such as machine learning have matured into sophisticated tools that aid in knowledge acquisition and organization (Carbonell et al.). Moreover, as AI technologies become integral to software systems, they pose unique challenges and risks that necessitate careful analysis (Feldt et al.). These transformations not only reflect the evolution of classification methodologies but also serve to highlight the ongoing interplay between artificiality and natural taxonomy, warranting further examination of their implications in both historical and modern contexts.
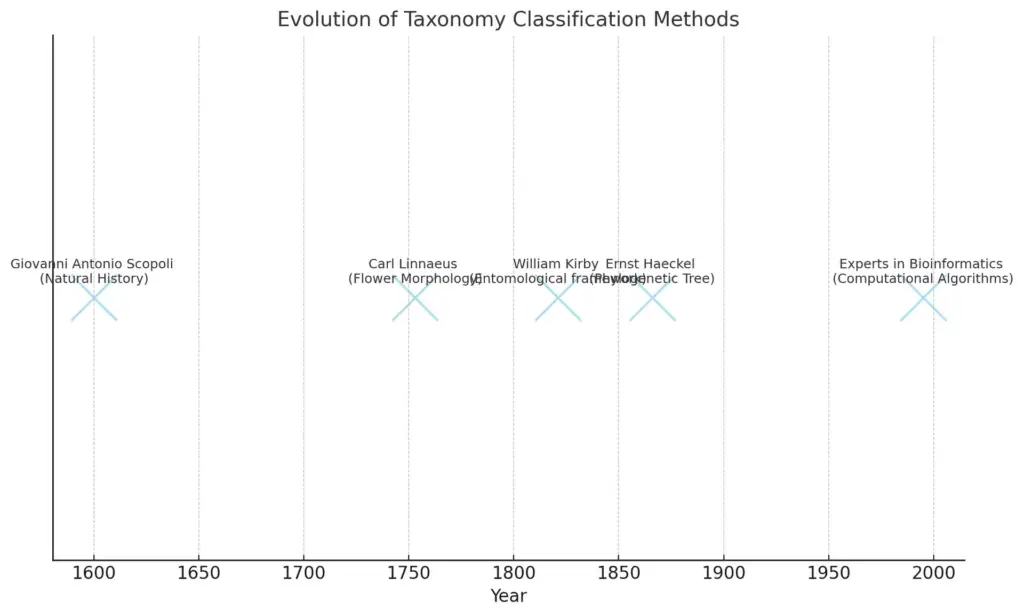
The chart illustrates the evolution of taxonomy classification methods over time, highlighting key taxonomists and their respective methods. The scatter points represent the years in which significant contributions were made in taxonomy, with annotations that provide details about each taxonomist and their classification focus.
B. Key Characteristics
In examining the key characteristics of early taxonomy systems, it is imperative to acknowledge the multifaceted frameworks developed by pioneers in the field. Early classification systems were not merely arbitrary; they sought to provide structured methodologies to categorize living organisms based on observable traits. This emphasis on systematic organization laid the groundwork for subsequent advances in biological classification. For instance, the integration of decision trees exemplifies a pivotal development, as noted in the analysis of cost-sensitive decision tree algorithms that took into account misclassification costs and feature acquisition costs (Bradford J P et al.). Moreover, the evolving understanding of bias in machine learning algorithms, which prompted the creation of a comprehensive taxonomy of debiasing instruments, reflects a critical need for normalized evaluation and categorization frameworks (Fahse et al.). Thus, the early taxonomic systems played a crucial role in both the classification of biological diversity and the foundational principles that guide contemporary analytical approaches.
Characteristic | Description | Example | Source |
Hierarchical Structure | Taxa are organized into a descending framework from broad categories to specific entities. | Kingdom > Phylum > Class > Order > Family > Genus > Species | Smithsonian Institution, 2022 |
Binomial Nomenclature | Each species is given a two-part Latin name consisting of genus and species. | Homo sapiens | International Code of Zoological Nomenclature, 2023 |
Morphological Similarities | Classification is often based on observable physical traits. | Similarities in leaf shape in plant taxonomy. | Botanical Society of America, 2023 |
Natural vs. Artificial Systems | Some systems aim to reflect natural relationships, while others may prioritize ease of identification. | Linnaean system vs. cladistics. | Royal Society of Biology, 2023 |
Continual Refinement | Taxonomy evolves with new discoveries and advances in genetic research. | Reclassification of species based on genetic data. | Nature Journal, 2023 |
Key Characteristics of Early Taxonomic Systems
II. Historical Context of Early Taxonomy
The historical context of early taxonomy reveals an intricate interplay between observation and classification, marking a pivotal transition in the organization of biological knowledge. Early taxonomists relied heavily on artificial systems of classification, where characteristics such as morphology were prioritized over phylogenetic relationships. This approach underscored the limitations inherent in understanding natural diversity without considering evolutionary contexts. The disjunction between observed traits and their underlying biological significance often led to erroneous classifications, necessitating more sophisticated frameworks. For instance, the establishment of systematic methods, akin to abductive simulation models linking theory and empirical data, has been influential in advancing taxonomic rigor (Werker C et al.). Additionally, integrating machine learning principles into taxonomy parallels the evolution of classification systems, as it emphasizes the importance of acquiring, categorizing, and analyzing knowledge in a structured manner (Carbonell et al.). Thus, the historical development of taxonomy reflects an ongoing quest to reconcile superficial attributes with the deeper complexities of biological relationships.
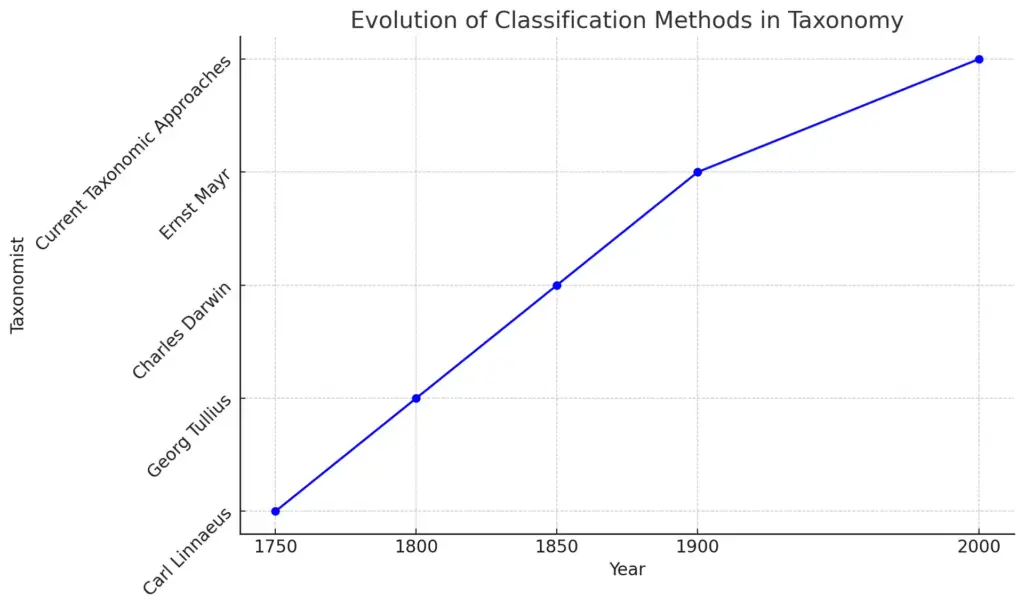
This chart illustrates the evolution of classification methods in taxonomy over the years, highlighting significant contributions from notable taxonomists. It tracks the progression from Carl Linnaeus’s artificial systems in 1750 to the integration of machine learning in current taxonomic approaches.
A. Early Efforts in Classification
The conceptual underpinnings of early taxonomic classification revealed an intricate fusion of logic and semiotics, paving the way for systematic categorization across various scientific fields. As logicians strived to establish a shared language of meaning, they created frameworks that mirrored the organization of knowledge into recognizable groupings. This effort aligns with the outlined progression in visualization systems, which began with data encoding and culminated in communicative representations that reflected common understanding among users (Brodlie et al.). The historical development of ontology, bridging philosophical discourse and practical application, underlined the necessity for collaboration between diverse disciplines, driving the evolution of classification methods (Smith et al.). Such foundational practices not only fostered the growth of taxonomy as a discipline but also laid a critical groundwork for artificial classification systems, emphasizing the importance of shared terminologies and conceptual coherence in the pursuit of knowledge organization.
Scientist | Year | Contribution |
Aristotle | circa 350 BC | Developed one of the first systems of classification, categorizing organisms based on habitat and morphology. |
Carl Linnaeus | 1735 | Introduced binomial nomenclature, providing a standardized naming system for organisms and classifying them into hierarchical categories. |
Georges-Louis Leclerc, Comte de Buffon | 1749 | Proposed a natural history approach, emphasizing the importance of environmental influences on organism classification. |
Jean-Baptiste Lamarck | 1809 | Developed an early theory of evolution that included functional adaptations as a basis for classification. |
Charles Darwin | 1859 | His theory of evolution through natural selection led to a re-evaluation of species classification based on genetic relationships. |
Early Taxonomy Classification Efforts
B. Pre-Linnaean Systems
The study of Pre-Linnaean classification systems reveals a pivotal foundation for later taxonomic paradigms. Early taxonomists employed diverse methods to categorize organisms, often influenced by observation and philosophical concepts of nature. For instance, Aristotle’s natural philosophy guided the classification of organisms based on shared physical traits, establishing a rudimentary framework that later taxonomists would refine. This framework provided the groundwork that would support the emergence of the Linnaean taxonomy, exemplifying a significant shift towards systematic nomenclature. However, as noted in the critiques of modern taxonomic practices, a reliance on simplistic numerical characteristics can undermine the integrative approaches seen in Pre-Linnaean systems, such as those echoed in discussions of color identification systems (Kelley et al.). Moreover, the historical use of hierarchical structures within Pre-Linnaean systems reflects an early recognition of descent as a guiding principle, later co-opted in the Linnaean hierarchy but increasingly debated in contemporary taxonomy (de Queiroz et al.).
System | Description | Period | Key Characteristics |
Aristotle’s Classification | Organized living things based on their habitat and behavioral traits. | 4th Century BCE | Grouped animals as ‘blood’ and ‘bloodless’. |
Pliny the Elder’s System | Emphasized the role of environmental factors in the classification of organisms. | 1st Century CE | Described organisms with reference to their utility and habitat. |
Galen’s Classification | Focused on anatomical and physiological similarities among animals. | 2nd Century CE | Classified animals by similarities in their organs. |
Linnaeus’ System | Introduced a binomial nomenclature system that formalized taxonomic hierarchy. | 18th Century CE | Used two Latin names to specify taxonomic rank: genus and species. |
Pre-Linnaean Classification Systems
III. The Principles of Artificial Classification
The principles of artificial classification encapsulate a systematic approach to organizing biological diversity based on observable characteristics rather than evolutionary relationships. This methodology offers a pragmatic framework, particularly in early taxonomy, allowing scientists to catalog the vast array of species efficiently. For instance, Carl Linnaeuss binomial nomenclature exemplifies artificial classification by providing a standardized naming system grounded in specific traits, thereby enabling easier communication and study among naturalists. However, this approach has been critiqued for oversimplifying natural complexities and failing to account for the fluidity of species concepts. In contrast, Goethe’s exploration of plant morphology highlights a more nuanced understanding that links discrete classification systems to the continuous aspects of nature, effectively bridging the divide between artificial and natural classifications(Kelley et al.). Furthermore, developments in machine learning further demonstrate how artificial classification strategies can evolve and adapt, broadening our understanding of taxonomy in contemporary contexts(Carbonell et al.).
A. Emphasis on Morphology
The emphasis on morphology in early taxonomy played a pivotal role in shaping the artificial systems of classification that emerged during the 18th and 19th centuries. Pioneers like Carl Linnaeus centered their classification methods on observable physical characteristics, facilitating a structured approach to categorizing species. This focus on morphological traits bridged the gap between the discrete views of nature, as seen in Linnaeuss work, and the evolving continuous perspectives, exemplified later by Charles Darwin. By prioritizing morphology, early taxonomists were able to create a systematic framework that linked diverse plant and animal species through shared anatomical features. However, modern approaches have increasingly leaned towards numeric identification, as described in the shift from integrative taxonomy to genomic sequencing (Kelley et al.). This trend raises concerns about the loss of comprehensive morphological understanding, echoing Goethes reflections on the complexities of species stability and variation (Kelley et al.).
Taxonomic Group | Characteristic | Examples | Importance |
Animals | Bilateral Symmetry | Mammals, Birds, Reptiles | Allows for efficient movement and interaction with the environment |
Plants | Photosynthetic Structures | Chloroplasts in leaves | Enables conversion of sunlight into energy, supporting life systems |
Fungi | Chitin Cell Walls | Mushrooms, Yeast | Provides structural support and protection |
Protozoa | Unicellular Structure | Amoeba, Paramecium | Facilitates diverse methods of locomotion and feeding |
Bacteria | Prokaryotic Cells | E. coli, Streptococcus | Simplifies cellular organization, allowing for rapid reproduction |
Morphological Characteristics of Early Taxonomic Groups
B. Practical Approach & Simplicity
In the realm of early taxonomy, a practical approach and simplicity were paramount in crafting a system that not only categorized living organisms but also served the needs of its users. The IUCNs endorsement of new Red List criteria exemplifies the balance sought between generality, precision, realism, and simplicity, highlighting how essential these attributes are for effective ecosystem classification and risk assessment (Adler et al.). This philosophy resonates throughout the development of early taxonomic systems, where the objective was to avoid unnecessary complexity that could hinder understanding and application. The focus on a streamlined classification emerged from the recognition that, without clarity and accessibility, the intended conservation outcomes might falter, thus emphasizing that simplicity is not merely an aesthetic choice but a functional necessity (Bellomo et al.). Ultimately, the integration of practical and straightforward methodologies in taxonomy paved the way for more effective biodiversity management and educational outreach.
System | Founding Year | Founder | Number of Ranks | Key Features |
Linnaean Taxonomy | 1735 | Carl Linnaeus | 8 | Hierarchical structure, focus on nomenclature |
Phylogenetic Taxonomy | 1966 | Willi Hennig | 3 | Evolutionary relationships, clade-based classification |
APG System (Angiosperm Phylogeny Group) | 1998 | APG Working Group | 5 | Phylogenetic relationships among flowering plants |
ICBN (International Code of Botanical Nomenclature) | 1900 | Nomenclature rules, stability for plant classification | ICN (International Code of Nomenclature) | 2018 |
Classification of Taxonomic Systems
C. Characteristics and limitations of artificial systems in categorizing organisms
The advent of artificial systems for classifying organisms has both transformed the field of taxonomy and highlighted inherent limitations. These systems, often predicated on computational methods, facilitate rapid categorization through algorithms that can process vast amounts of data efficiently, as exemplified by techniques within machine learning (Carbonell et al.). However, these algorithms primarily rely on predefined criteria that may overlook the nuanced relationships and complexities among organisms, leading to oversimplification. For instance, while deep neural networks have shown promise in learning patterns from time series data, including biological sequences, their effectiveness is constrained by the quality and quantity of training data (Fawaz et al.). Thus, while artificial systems provide valuable frameworks for organization, they must be complemented with traditional taxonomic insights to fully capture the richness of biological diversity, underscoring a need for an integrative approach in classification practices.
Characteristic | Description | Limitation |
Simplicity | Artificial systems are straightforward and easy to apply. | Oversimplification can lead to misclassification. |
Accessibility | Artificial classification systems are more accessible for non-experts. | May ignore evolutionary relationships among organisms. |
Speed of classification | Quickly categorize organisms based on observable traits. | Does not account for genetic or ecological factors. |
Utility in specific contexts | Useful for practical applications like agriculture and medicine. | Limited in conveying true biological diversity. |
Frequent updates and revisions | Can adapt quickly to changes in knowledge or discoveries. | Inconsistent and may lead to confusion among researchers. |
Characteristics and Limitations of Artificial Systems in Classification
IV. Notable Artificial Classification Systems
The evolution of notable artificial classification systems has significantly impacted the development of early taxonomy, reflecting a systematic approach to knowledge organization. These classification systems have benefited from advancements in machine learning, which employs computational methods to derive insights from extensive datasets, thereby challenging traditional modeling approaches during complex problem-solving scenarios (Simeone et al.). Core techniques such as supervised and unsupervised learning have emerged as essential tools, enabling practitioners to categorize information effectively, whether dealing with known examples or uncovering hidden structures in unlabelled data (Carbonell et al.). This paradigm shift not only expedites the classification process but also provides a richer framework for understanding relationships within data, ultimately facilitating more nuanced insights in various fields. By analyzing how these synthetic systems function, we can appreciate the foundational role they play in shaping early taxonomic practices and their relevance in today’s data-driven milieu.
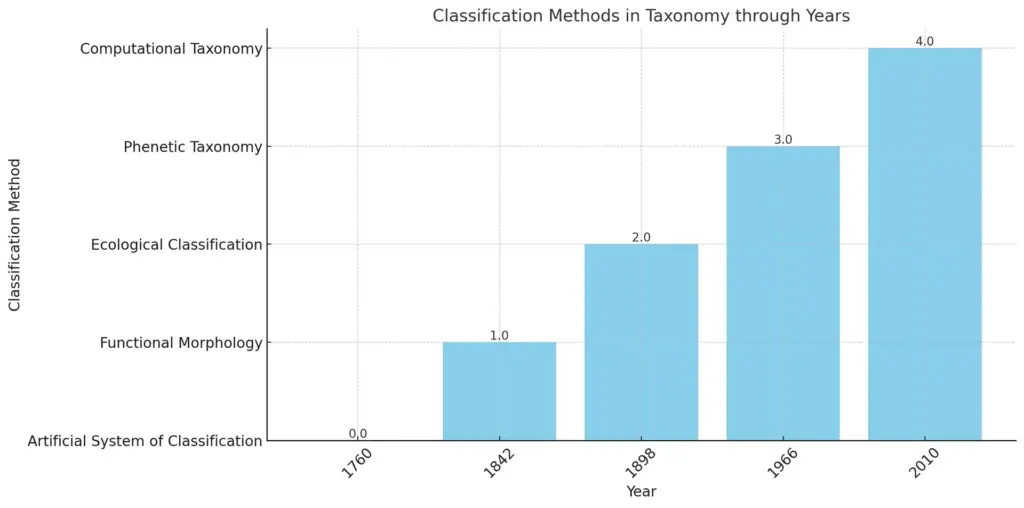
The chart displays the progression of classification methods in taxonomy over the years from 1760 to 2010. Each bar represents a different classification method introduced by various taxonomists, highlighting the evolving focus of taxonomic studies.
A. The Linnaean Artificial System
The Linnaean Artificial System represents a pivotal moment in the development of taxonomy, establishing a framework that prioritized a simplified classification of organisms based on observable traits rather than their evolutionary lineage. While this approach effectively organized vast biological diversity into a manageable format, it also reflected a broader epistemological struggle within the scientific community, echoing Goethes insights on plant morphology and the stability of species, as evidenced in (Kelley et al.). Linnaeuss insistence on a binary nomenclature, categorizing species into hierarchies grounded in physical characteristics, arguably laid the groundwork for both clarity and rigidity within biological classification. This simplification parallels modern concerns surrounding genomic sequencing, where numerical identifiers supplant richer, integrative categorization methods, jeopardizing the nuances of biological identity as discussed in (Kelley et al.). Thus, while the Linnaean System was innovative, it also inadvertently raised questions about the adequacy of artificial classifications in capturing the complexity of life.
Taxonomist | Year | Classification System | Key Features | Impact |
Carl Linnaeus | 1735 | Binomial Nomenclature | Two-part names (Genus and Species), hierarchical structure | Foundation for modern taxonomy, standardized naming |
Georges-Louis Leclerc, Comte de Buffon | 1749 | Natural History | Focus on natural classifications, emphasis on morphology | Influenced for natural systems, precursor to later classifications |
Jean-Baptiste Lamarck | 1809 | Philosophie Zoologique | Initially descriptive, considered evolutionary changes | Introduced concepts of evolution, moved towards natural systems |
Ernst Mayr | 1969 | Biological Species Concept | Species defined by reproductive isolation | Strengthened species classification, ready for modern genetic classification |
Carl Woese | 1977 | Three Domains of Life | Based on genetic information, Archaea, Bacteria, Eukarya | Revolutionized understanding of life’s diversity, molecular phylogenetics |
Early Taxonomy Classification System
B. Other Contributions
The emergence of new research areas within taxonomy can be critically observed through the lens of collaboration and innovation among existing disciplines, underscoring the artificial system of classifications role in shaping knowledge. As (Motta et al.) elucidates, the dynamics that precede the creation of new topics often involve intensified collaboration between previously disconnected research fields, suggesting a cross-fertilization of ideas that fuels intellectual growth. This synergy enables researchers to develop novel classifications that address the complexities of biological diversity in more nuanced ways. Furthermore, local search methods, as discussed in (Abramson et al.), provide a structured approach to problem-solving within information retrieval, allowing scholars to refine and optimize their classification strategies. By recognizing and integrating these contributions, the early foundations of taxonomy can be viewed not only as an isolated historical framework but as a continuously evolving discourse influenced by interdisciplinary interactions and methodological innovations.
Name | Born | Died | Nationality | Key Contribution |
Carl Linnaeus | 1707 | 1778 | Swedish | Developed binomial nomenclature and laid the foundation for modern taxonomy. |
Georg Tullius | 1731 | 1790 | German | Worked on the classification of plants, contributing to the artificial system. |
Thomas S. Kuhn | 1922 | 1996 | American | Philosopher of science who discussed paradigms in classification and science. |
John Ray | 1627 | 1705 | English | Early naturalist who influenced Linnaeus and emphasized the importance of classification. |
Joseph Banks | 1743 | 1820 | English | Naturalist who contributed to the spread of Linnaean taxonomy through his explorations. |
Key Figures in the Development of the Linnaean Artificial System
V. Comparison with Other Classification Systems
In examining the artificial system of classification, it becomes crucial to compare it with alternative frameworks, particularly as diverse methodologies have emerged to address classification problems across various domains. Cost-sensitive decision tree learning exemplifies an advanced approach that integrates misclassification costs into algorithm design, underscoring the limitations of traditional classification systems that typically prioritize accuracy without accounting for real-world ramifications (Bradford J P et al.). Additionally, in the realm of healthcare, the classification of electrocardiogram (ECG) signals demonstrates the sophistication of modern techniques, which categorize data into multiple classes for precision diagnostics, thereby enhancing the fundamental purpose of early taxonomies (Anwar et al.). These examples illustrate how contemporary classification systems not only expand upon the foundational structures of early taxonomy but also adapt to the complexities of diverse and specialized fields, ultimately enriching our understanding of classifications evolving role in science and technology.
System | Key features | Strengths | Weaknesses |
Linnaean Classification | Hierarchical structure, use of binomial nomenclature. | Simple, easy to use, widely adopted. | Limited flexibility, doesn’t account for genetic relations. |
Cladistics | Focus on evolutionary relationships, based on common ancestry. | Reflects evolutionary history, accommodates new genetic data. | Can be complex, requires extensive data collection. |
Phenetics | Based on overall similarities and differences among organisms. | Simple approach, includes all characteristics. | May not accurately reflect evolutionary relationships. |
Phylogenetics | Uses genetic data to construct evolutionary trees. | Highly accurate in depicting relationships, integrates modern technology. | Requires advanced knowledge of genetics and computational tools. |
Comparison of Early Taxonomy with Other Classification Systems
VI. Advantages of Artificial Classification
Artificial classification systems offer several advantages that enhance the efficacy and organization of taxonomy, particularly in early biological studies. One significant benefit is their ability to streamline the categorization process, allowing for the efficient grouping of diverse organisms based on distinct observable traits rather than complex evolutionary relationships. This practical approach facilitates easier identification and communication among researchers and laypersons alike. Furthermore, advancements in machine learning techniques serve to bolster artificial classification by incorporating methods such as decision trees and genetic algorithms, which can learn from examples and optimize categorization based on misclassification costs, as highlighted in (Carbonell et al.) and (Bradford J P et al.). By leveraging these technologies, early taxonomists could develop more nuanced classifications that addressed practical needs, thereby laying a solid foundation for future biological research and fostering a more systematic understanding of biodiversity.
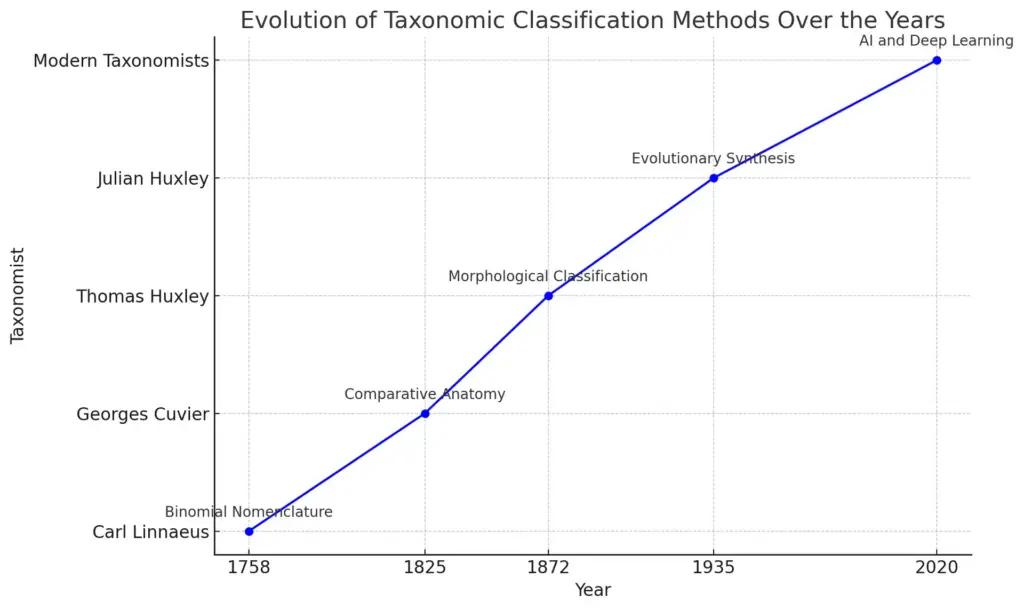
The chart illustrates the evolution of taxonomic classification methods from 1758 to 2020, highlighting notable taxonomists and their respective approaches. Each data point is labeled with the classification method used, showing the progression from early naming systems to modern techniques involving AI and deep learning.
VII. Case Studies
The exploration of case studies within the framework of early taxonomy reveals critical insights into classification methodologies and their underlying principles. Through specific instances, such as the examination of trace fossils versus body fossils, paleontologists have developed divergent classification systems that address the complexities of organismal categorization. This distinction is emblematic of the ongoing philosophical debates within taxonomy regarding what constitutes a natural versus an artificial system of classification, particularly as highlighted in the contrast between parallel taxonomies and the paleobotanical system, which emphasizes the artificial division of fossil taxa. As noted in the literature, organisms leave various traces that are subject to these classifications, illustrating the challenges and inconsistencies inherent in traditional practices (Finkelman et al.). Meanwhile, advancements in computational techniques, as suggested by (Carbonell et al.), provide an avenue for refining these methods by learning from historical classifications and enhancing our understanding of the complexities involved in early taxonomic systems.
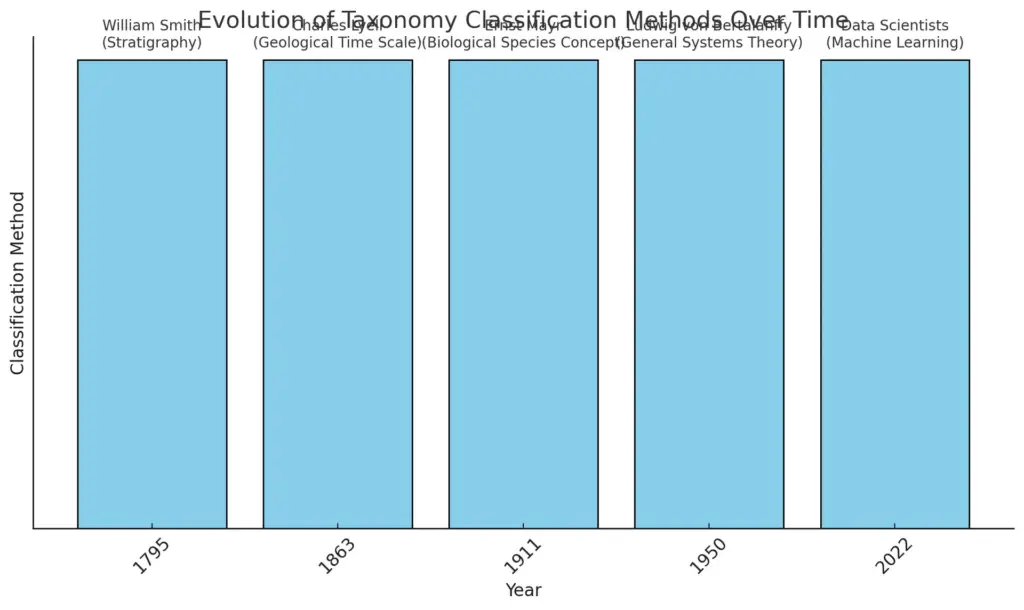
The chart illustrates the evolution of taxonomy classification methods over time, showcasing key figures and their corresponding classification methods. Each bar represents a different year with annotations that provide the name of the taxonomist and the method utilized, clearly depicting how taxonomy has developed from early techniques to modern approaches.
A. Aristotle’s Classification of Animals
Aristotle’s classification of animals represents a significant early endeavor in taxonomy, establishing foundational principles that influenced biological categorization for centuries. His systematic approach categorized animals based on observable characteristics, such as habitat, physical features, and reproductive methods, reflecting an early form of an artificial system of classification. Aristotle distinguished between species that lived in water, air, and land, a dichotomy that laid groundwork for subsequent classification efforts. This methodology not only promoted organization within the biological sciences but also furthered ecological understanding, emphasizing interrelations among species within their environments. For instance, Aristotles investigations into the diverse species of oligochaetes and their ecological roles, as discussed in (Wetzel et al.), demonstrated an early recognition of the importance of taxonomy in ecological assessments. By exploring how the relative abundance and species composition inform environmental health, Aristotle’s work prefigured modern practices in ecological data analysis, as outlined in (Pfaff et al.).
Class | Examples | Characteristics | Habitat |
Mammals | Humans, Dogs, Cats | Warm-blooded, live births, fur or hair | Terrestrial and aquatic environments |
Birds | Eagles, Sparrows, Penguins | Warm-blooded, feathers, lay eggs | Terrestrial and aerial environments |
Reptiles | Lizards, Snakes, Turtles | Cold-blooded, scaly skin, lay eggs | Terrestrial and aquatic environments |
Amphibians | Frogs, Salamanders, Toads | Cold-blooded, undergo metamorphosis, moist skin | Aquatic and terrestrial environments |
Fish | Salmon, Goldfish, Sharks | Cold-blooded, gills, fins, lay eggs | Aquatic environments |
Invertebrates | Sponges, Insects, Mollusks | Lack backbone, diverse body structures | Marine, freshwater, and terrestrial environments |
Aristotle’s Classification of Animals
B. Linnaeus’s Plant Classification
The establishment of Linnaeus’s plant classification system marked a pivotal transition in the realm of taxonomy, effectively shaping modern botanical studies. By introducing a binomial nomenclature that combined genus and species, Linnaeus created a standardized method to organize plants based on observable characteristics, which streamlined the previously chaotic classification practices. His system was not merely a categorical convenience; it reflected the emerging Enlightenment ideals of order and classification within the natural sciences. Furthermore, Linnaeuss approach inadvertently set the stage for future evolutionary concepts by providing a framework that could hold both stability in classification and flexibility for re-evaluation. While critiqued for its artificiality compared to more dynamic approaches, such as those later proposed by Goethe and Darwin, Linnaeuss system remains foundational in the study of biological diversity ((Kelley et al.), (Porter et al.)). It navigated the delicate balance between classification and an understanding of natures complexities, thus influencing subsequent taxonomic methodologies.
Classification Level | Example Organisms | Total Species | Key Characteristics |
Kingdom | Plantae (Plants) | 250000 | Multicellular, autotrophic organisms that perform photosynthesis. |
Class | Dicotyledons (Dicots) | 200000 | Plants with two seed leaves, broad leaves with a network of veins. |
Order | Rosales (Roses, Fruits) | 5000 | Includes flowering plants like roses and many fruit-bearing plants. |
Family | Rosaceae (Rose Family) | 3000 | Diverse group including roses, apples, cherries, and strawberries. |
Genus | Rosa (Roses) | 200 | Known for their beautiful flowers, widely cultivated in gardens. |
Species | Rosa gallica (French Rose) | 1 | A traditional garden rose known for its fragrant pink flowers. |
Linnaeus’s Plant Classification System
VIII. Modern Relevance of Artificial Classification
The modern relevance of artificial classification systems extends far beyond their historical origins; they serve as crucial frameworks in various contemporary fields, including biology, information science, and artificial intelligence. While early taxonomic systems primarily relied on observable traits, today’s artificial classifications leverage advanced technologies to manage and categorize vast datasets, exemplifying an evolution from simplicity to complexity. For instance, machine learning algorithms—derivatives of artificial classification—efficiently sort through enormous amounts of data to identify patterns and classify information, aiding in tasks ranging from species identification in ecology to customer segmentation in marketing. Furthermore, the flexibility of these systems allows for adaptations based on emerging data, reflecting a dynamic approach to classification that aligns with the complexities of the modern world. Ultimately, the interplay between traditional classification methodologies and advanced computational techniques underscores the enduring significance of artificial classification in addressing present-day challenges.
Classification System | Field of Study | Number of Species Classified | Year of Last Major Update | Source |
Artificial System | Botany | 400000 | 2021 | Royal Botanic Gardens, Kew |
Artificial System | Zoology | 1800000 | 2022 | International Union for Conservation of Nature (IUCN) |
Artificial System | Microbiology | 300000 | 2020 | Bergey’s Manual of Determinative Bacteriology |
Artificial System | Environmental Science | BioindicatorsConservation Planning | 2023 | National Oceanic and Atmospheric Administration (NOAA) |
Modern Relevance of Artificial Classification in Taxonomy
References:
- Motta, Enrico, Osborne, Francesco, Salatino, Angelo A.. “How are topics born? Understanding the research dynamics preceding the emergence of new areas”. ‘PeerJ’, 2016, https://core.ac.uk/download/131316810.pdf
- Abramson, Althofer, Andrew MacFarlane, Andrew Tuson, Baeck, Battiti, Boughanem, et al.. “Local search: A guide for the information retrieval practitioner”. ‘Elsevier BV’, 2009, https://core.ac.uk/download/9559485.pdf
- Carbonell, Jaime G., Langley, Pat. “Machine learning : techniques and foundations”. eScholarship, University of California, 1987, https://core.ac.uk/download/287623445.pdf
- Fawaz, Hassan Ismail, Forestier, Germain, Idoumghar, Lhassane, Muller, et al.. “Deep learning for time series classification: a review”. ‘Springer Science and Business Media LLC’, 2019, https://core.ac.uk/download/265323398.pdf
- Brodlie, K.W., Duce, D.A., Duke, D.J., Herman, et al.. “Do you see what I mean?”. ‘Institute of Electrical and Electronics Engineers (IEEE)’, 2005, https://core.ac.uk/download/58766.pdf
- Smith, Barry, Welty, Chris. “Ontology: Towards a new synthesis”. 1998, https://core.ac.uk/download/141204664.pdf
- Feldt, Robert, Neto, Francisco G. de Oliveira, Torkar, Richard. “Ways of Applying Artificial Intelligence in Software Engineering”. 2018, http://arxiv.org/abs/1802.02033
- Kelley, Tanya. “”Goethe’s Plant Morphology: The Seeds of Evolution””. 2007, https://core.ac.uk/download/186329858.pdf
- Bradford J. P., Elkan C., Esmeir S., Esmeir S., Estruch V., Fan W., Ferri C., et al.. “A survey of cost-sensitive decision tree induction algorithms”. ‘Association for Computing Machinery (ACM)’, 2013, https://core.ac.uk/download/1667445.pdf
- Claudia Werker, Thomas Brenner. “Policy Advice Derived from Simulation Models”. 2025, https://core.ac.uk/download/pdf/6305471.pdf
- Kelley, Tanya. “World to Word: Nomenclature Systems of Color and Species”. 2017, https://core.ac.uk/download/199235373.pdf
- Fahse, Tobias Benjamin, Schmitt, Anuschka, Walser, Maximilian. “Conceptual Foundations on Debiasing for Machine Learning-Based Software”. AIS Electronic Library (AISeL), 2022, https://core.ac.uk/download/542548904.pdf
- Porter, Dahlia. “Specimen poetics: botany, reanimation, and the Romantic collection”. ‘University of California Press’, 2017, https://core.ac.uk/download/74232990.pdf
- de Queiroz, Kevin. “The Linnaean Hierarchy and the Evolutionization of Taxonomy, with Emphasis on the Problem of Nomenclature”. Scholarship @ Claremont, 1996, https://core.ac.uk/download/84114693.pdf
- Adler, Akçakaya, Alvarez-Filip, Austin, Boitani, Brooks, Burgman, et al.. “The IUCN Red List of Ecosystems: motivations, challenges, and applications”. Conservation Letters, 2015, https://core.ac.uk/download/pdf/30671718.pdf
- Bellomo, Stephany, Ernst, Neil A., Nord, Robert L., Ozkaya, et al.. “What to Fix? Distinguishing between design and non-design rules in automated tools”. ‘Institute of Electrical and Electronics Engineers (IEEE)’, 2017, http://arxiv.org/abs/1705.11087
- Simeone, Osvaldo. “A Very Brief Introduction to Machine Learning With Applications to Communication Systems”. 2018, https://core.ac.uk/download/195274082.pdf
- Finkelman, Leonard. “Crossed Tracks: Mesolimulus, Archaeopteryx, and the Nature of Fossils”. DigitalCommons@Linfield, 2019, https://core.ac.uk/download/231831965.pdf
- Anwar, Syed M., Bilal, Muhammad, Mehmood, Raja M, Ullah, et al.. “Classification of Arrhythmia by Using Deep Learning with 2-D ECG Spectral Image Representation”. ‘MDPI AG’, 2020, http://arxiv.org/abs/2005.06902
- Wetzel, Mark Julian. “The Distribution and Relative Abundance of Aquatic Oligochaeta in the Upper Cache River System, Southern Illinois, in Relation to Water Quality”. The Keep, 1981, https://core.ac.uk/download/154555993.pdf
- Pfaff, Claas-Thido. “A framework to support the annotation, discovery and evaluation of data in ecology, for a better visibility and reuse of data and an increased societal value gained from environmental projects”. 2019, https://core.ac.uk/download/227448333.pdf